VDTuner: A Machine Learning-Based Automatic Performance Tuning Framework for Vector Data Management Systems (VDMSs)
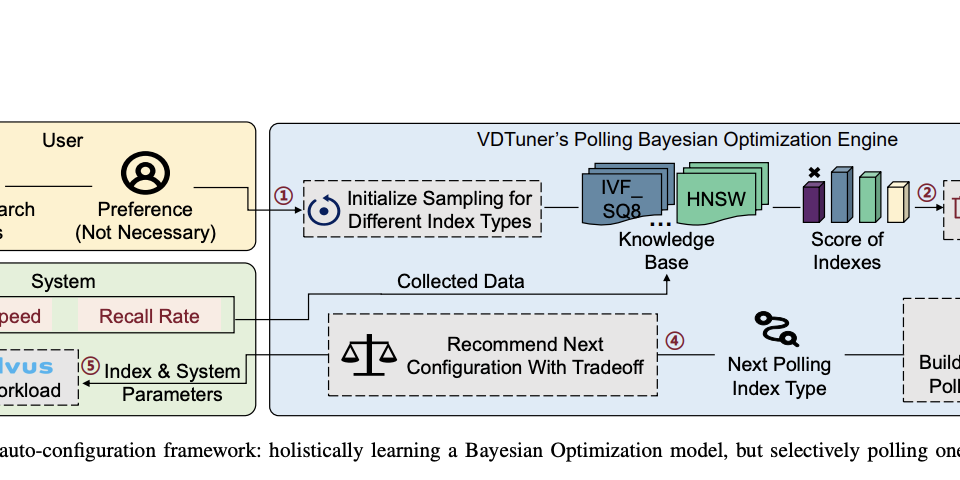
Large Language Models (LLMs) have steered in a period of extraordinary growth for Artificial Intelligence (AI) technology. To address issues like conversation hallucination, these models are being used increasingly in various settings where unstructured multimedia data is converted into embedding vectors. Vector Data Management Systems (VDMSs) are specifically designed to manage these vectors effectively. Platforms, such as Qdrant and Milvus, have developed sizable user bases and vibrant communities, serving as the backbone of the LLM age.
LLMs and other machine learning and information retrieval systems depend heavily on Vector Data Management Systems. These systems rely on effective similarity search, which is made possible by VDMSs, which provide users with the ability to define many adjustable indexes and system parameters. Nonetheless, the intrinsic intricacy of VDMSs presents noteworthy obstacles for automated performance optimization, which current techniques find difficult to tackle sufficiently.
In recent research, a team of researchers has presented VDTuner, a learning-based automatic performance tuning framework created especially for VDMSs, as a solution to these problems. Without requiring users to know anything beforehand, VDTuner effectively navigates the complex multi-dimensional parameter space of VDMSs by utilizing multi-objective Bayesian optimization. It also strikes a delicate balance between recall rate and search speed, producing an ideal configuration that improves performance overall.
The team has shared that various assessments have shown that VDTuner is effective. When compared to default settings, it significantly enhances VDMS performance, increasing search speed by 14.12% and recall rate by 186.38%. VDTuner achieves up to 3.57 times faster-tuning efficiency compared to the latest baselines. It provides scalability to meet individual user preferences and optimize budget-conscious goals.
The team has summarized their primary contribution as follows.
- To identify the main difficulties in fine-tuning Vector Data Management Systems, extensive exploratory research has been carried out. The team has examined the drawbacks of current VDMS tuning options, offering a thorough grasp of the state of the field at the moment.
- VDTuner has been introduced, which is a unique framework for performance tuning designed for VDMS. By utilizing Multi-objective Bayesian Optimisation, VDTuner effectively explores the intricate parameter space of VDMS in order to identify the ideal setup. This strategy seeks to fulfill a crucial demand in VDMS optimization by optimizing search speed and recall rate at the same time.
- To confirm VDTuner’s effectiveness, thorough assessments have been conducted which show through extensive testing that VDTuner performs far better than all current baselines. An in-depth research has also been carried out to comprehend the elements influencing its effectiveness, offering perceptions of its exceptional performance.
In conclusion, VDTuner is a big step forward in automatically adjusting VDMS performance and gives users a strong tool to improve the effectiveness and efficiency of their systems.
Check out the Paper. All credit for this research goes to the researchers of this project. Also, don’t forget to follow us on Twitter. Join our Telegram Channel, Discord Channel, and LinkedIn Group.
If you like our work, you will love our newsletter..
Don’t Forget to join our 40k+ ML SubReddit
Tanya Malhotra is a final year undergrad from the University of Petroleum & Energy Studies, Dehradun, pursuing BTech in Computer Science Engineering with a specialization in Artificial Intelligence and Machine Learning.
She is a Data Science enthusiast with good analytical and critical thinking, along with an ardent interest in acquiring new skills, leading groups, and managing work in an organized manner.