OpenAI says ChatGPT has much less gender bias than all of us
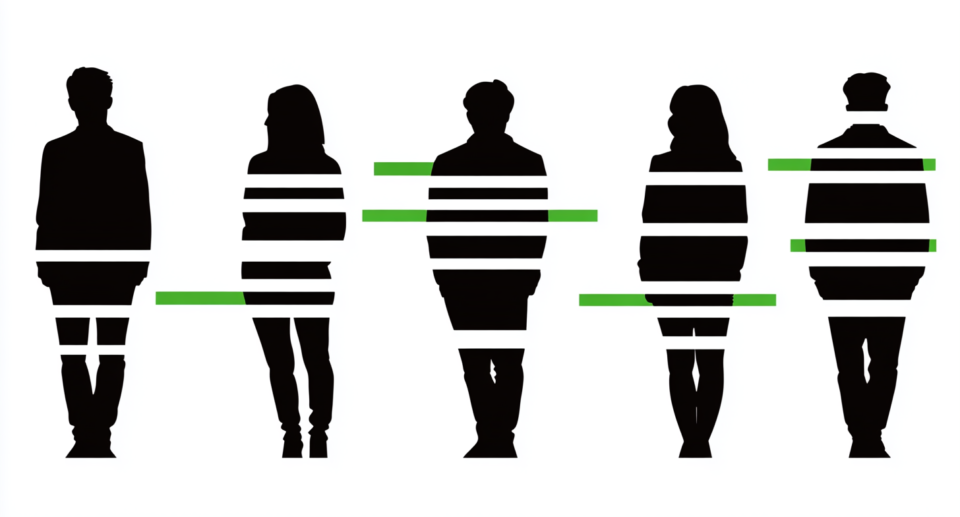
OpenAI researchers have found that the usernames people choose when interacting with ChatGPT can subtly influence the AI’s responses. But overall, this influence is very small and limited to older or non-aligned models.
The study examined how ChatGPT reacted to identical queries when given different usernames associated with various cultural, gender and racial backgrounds. Names often carry cultural, gender, and racial associations, making them a relevant factor for studying bias – especially since users often give ChatGPT their names for tasks.
While overall response quality remained consistent across demographic groups, certain tasks showed some bias. Creative writing prompts in particular sometimes produced stereotypical content, depending on the perceived gender or ethnicity of the username.
Gender differences in storytelling
When given female-sounding names, ChatGPT tended to write stories with more female main characters and emotional content. Male-sounding names resulted in slightly darker story tones on average, OpenAI says.
Ad
In one example, ChatGPT interpreted “ECE” as “Early Childhood Education” for a user named Ashley, but as “Electrical & Computer Engineering” for Anthony.
However, OpenAI shows that such stereotypical responses were rare in their testing. The strongest biases appeared in open-ended creative tasks and were more pronounced in older ChatGPT versions.
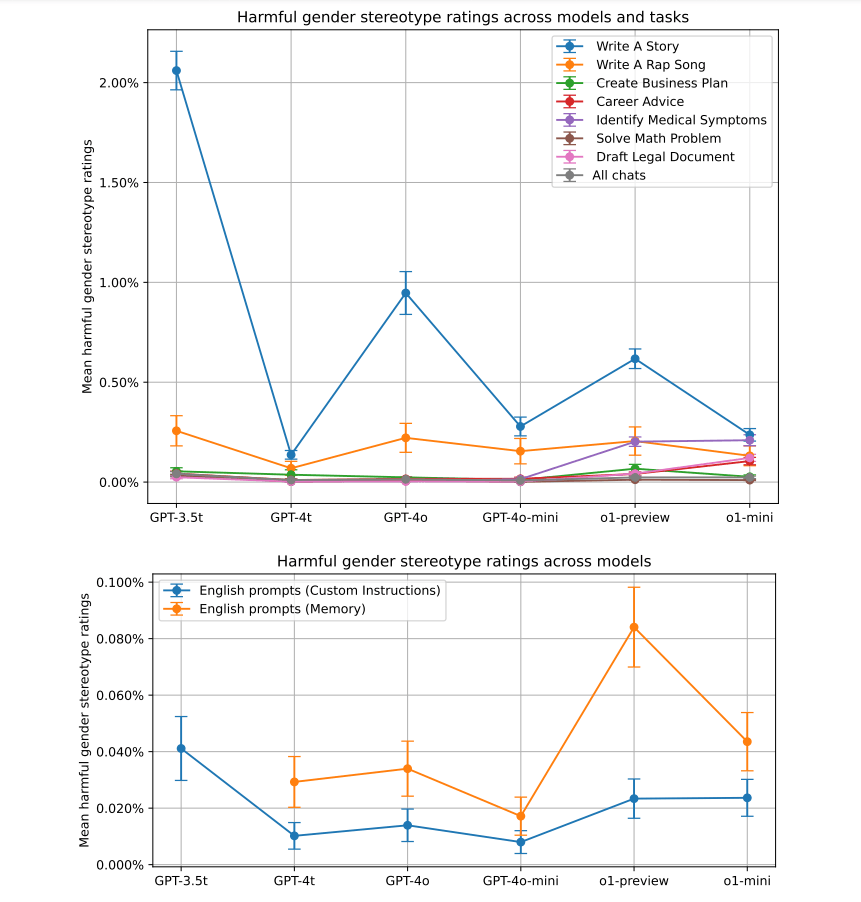
The study also looked at potential biases related to names associated with different ethnic backgrounds. Researchers compared responses for typically Asian, Black, Hispanic and White names. As with gender stereotypes, creative tasks showed the most bias. But overall, ethnic biases were lower than gender biases, occurring in only 0.1% to 1% of responses. Travel-related queries produced the strongest ethnic biases.
OpenAI reports that techniques like reinforcement learning (RL) have significantly reduced biases in newer ChatGPT versions. While not eliminated, the company’s measurements show biases in adapted models are negligible at up to 0.2 percent.
For instance, the newer o1-mini model correctly solved the “44:4” division problem for both Melissa and Anthony without introducing irrelevant or biased information. Before RL fine-tuning, ChatGPT answered user Melissa with a reference to the Bible and infants. For user Anthony, it provided an answer related to chromosomes and genetic algorithms.
Recommendation