How the US predicted Taliban attacks in Afghanistan
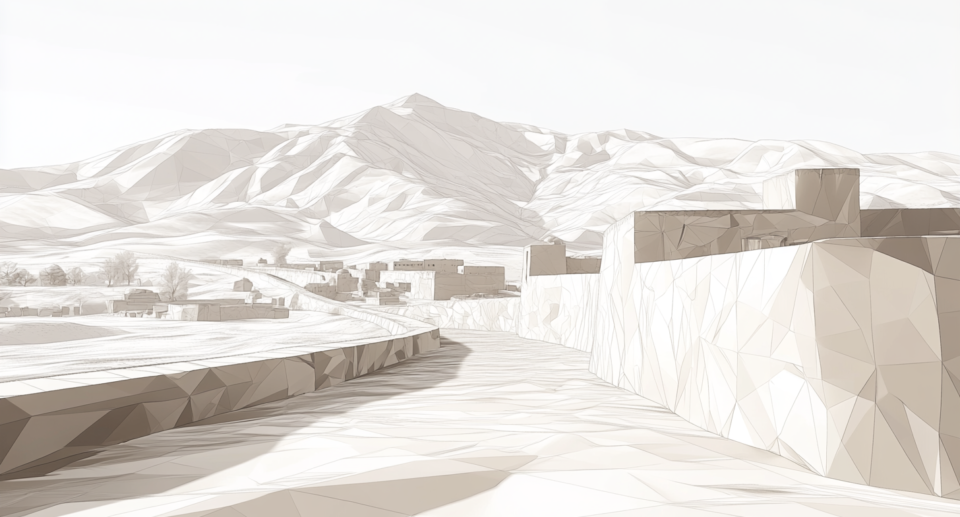
Under the pressure of dwindling resources and increasing violence in Afghanistan, the US military developed an AI system in 2019 to predict Taliban attacks. It was surprisingly accurate.
As NATO forces gradually reduced their troop strength in Afghanistan in 2019, the US military faced the challenge of conducting its intelligence work with fewer resources. Under the pressure of increasing Taliban attacks, a small team of intelligence officers launched the AI project “Raven Sentry.”
In October 2019, the team affectionately called the “nerd locker” began developing Raven Sentry. The AI model was designed to assess the risk of attacks on district or provincial centers and estimate the number of potential casualties using data from open sources such as weather reports, social media posts, news, and commercial satellite images.
The team first examined recurring patterns in insurgent attacks dating back to the Soviet occupation of Afghanistan in the 1980s. “In some cases, modern attacks occurred in the exact locations, with similar insurgent composition, during the same calendar period, and with identical weapons to their 1980s Russian counterparts.,” writes Colonel Thomas Spahr, who led the experiment, in an article for the US Army War College’s Parameters journal.
Ad
The “nerd locker” team was embedded in a special unit whose culture was better suited for free experimentation. The analysts were required to take regular shifts in the operations center to develop an understanding of mission requirements and build trust: “Trust in the people running the system led to trust in the system’s output,” says Colonel Spahr.
Fewer attacks in cold weather
Silicon Valley experts helped develop a neural network that correlated historical attack data with a variety of open sources.
In a first step, OSINT (Open-Source Intelligence) data, i.e., data from publicly accessible sources such as newspapers or social networks, had to be made machine-readable. Additionally, the analysts deconstructed historical events into individual components and labeled them for the system.
In a second step additional indicators, such as surrounding activities in mosques, madrassas, insurgent routes, and known meeting points were collected. So-called “influence data sets” with factors on weather conditions and political stability were also included. For example, according to Raven Sentry, attacks were more likely when the temperature was above 4°C, the moon brightness was below 30 percent, and it was not raining.
The Raven Sentry prototype was finally trained on three declassified databases of historical attacks and monitored 17 commercial geodata sources, OSINT reports, and GIS (Global Information Systems) datasets.
Recommendation
Once a certain combination of factors reached a threshold, the system triggered a warning. Reports of political or military gatherings, for example, drew the system’s attention. Movement patterns along historical insurgent infiltration routes could also trigger warning signals for the region.
Multiple anomalies were usually required to exceed the risk threshold and trigger a warning. For certain regions, known as warning named areas of interest (WNAIs), the risk was then increased and measures could be taken in consultation with the human analysts.
Human performance levels
By October 2020, the model had achieved an accuracy of 70 percent, similar to the performance of human analysts, “just at a much higher rate of speed,” Spahr told The Economist. The analysts did not treat the results as infallible but used them to deploy classified systems such as spy satellites or intercepted communications more precisely.
Raven Sentry was “learning on its own,” and “getting better and better by the time it shut down” says Colonel Spahr. In the relatively short time of operation, the system had gathered valuable experience on how AI systems can support analysts in processing and reviewing large amounts of sensor data.
In the three years since the discontinuation of Raven Sentry, military and intelligence agencies have invested many resources in AI-assisted early detection of attacks. “If we’d have had these algorithms in the run-up to the Russian invasion of Ukraine, things would have been much easier,” a source from British Defence Intelligence told The Economist.
But Colonel Spahr emphasizes the limitations of the system: “Just as Iraqi insurgents learned that burning tires in the streets degraded US aircraft optics or as Vietnamese guerrillas dug tunnels to avoid overhead observation, America’s adversaries will learn to trick AI systems and corrupt data inputs.” After all, the Taliban had gained the upper hand in Afghanistan despite the advanced technology of the US and NATO.
“Raven Sentry made the analysts more efficient but could not replace them,” Colonel Spahr summarizes the success of the experiment. After the first real-world experiences, urgent questions arise about the future applications of AI in warfare: “As the speed of warfare increases and adversaries adopt AI, the US military may be forced to move to an on-the-loop position, monitoring and checking outputs but allowing the machine to make predictions and perhaps order action,” says Colonel Spahr.