Deepseek’s $5.6M Chinese LLM wonder shakes up the AI elite
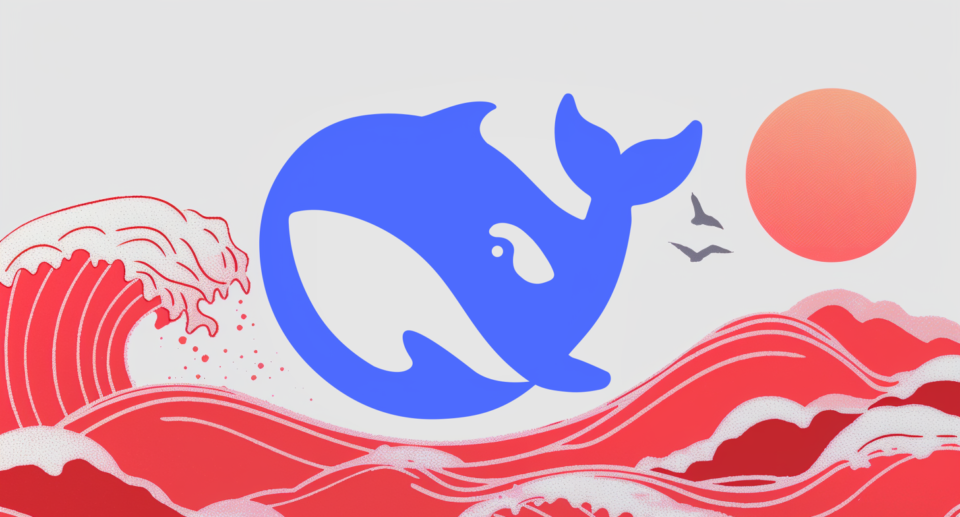
A Chinese startup is proving you don’t need deep pockets to build world-class AI. Deepseek’s latest language model goes head-to-head with tech giants like Google and OpenAI – and they built it for a fraction of the usual cost.
According to independent testing firm Artificial Analysis, Deepseek’s new V3 model can compete with the world’s most advanced AI systems, with a total training cost of just $5.6 million. That’s remarkably low for a model of this caliber.
In Artificial Analysis‘ comprehensive Quality Index, which combines results from various benchmarks, Deepseek-V3 scored 80 points. This puts it in the top tier alongside industry heavyweights like Gemini 1.5 Pro and Claude Sonnet 3.5. While Google’s Gemini and OpenAI’s latest models still lead the pack, Deepseek-V3 has surpassed every other open-source model available today.
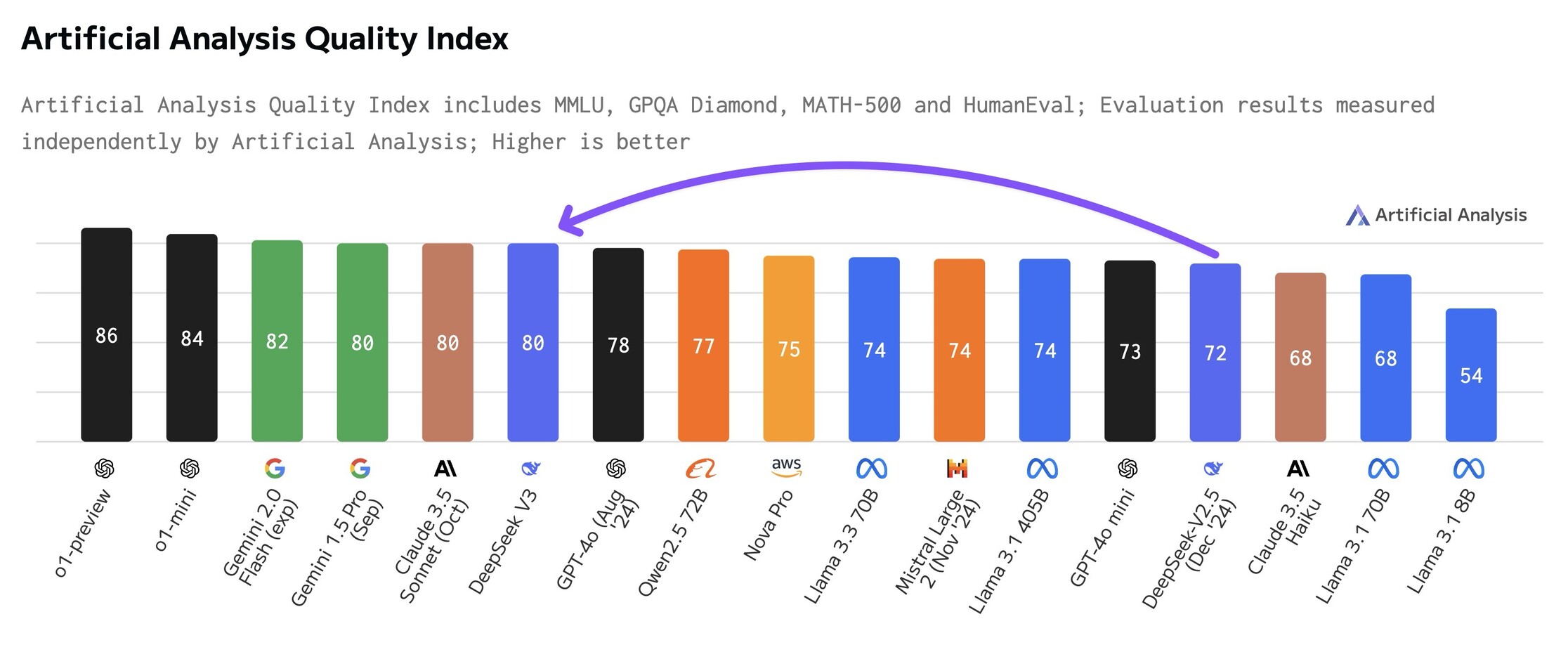
The model really shines at technical tasks. It scored an impressive 92% on the HumanEval programming test and demonstrated strong mathematical abilities with an 85% score on the MATH 500 challenge. These capabilities build on Deepseek’s earlier work with their R1 reasoning model from late November, which helped enhance V3’s problem-solving skills. Even Meta’s chief AI researcher, Yann LeCun, took notice, calling the model “excellent.”
Ad
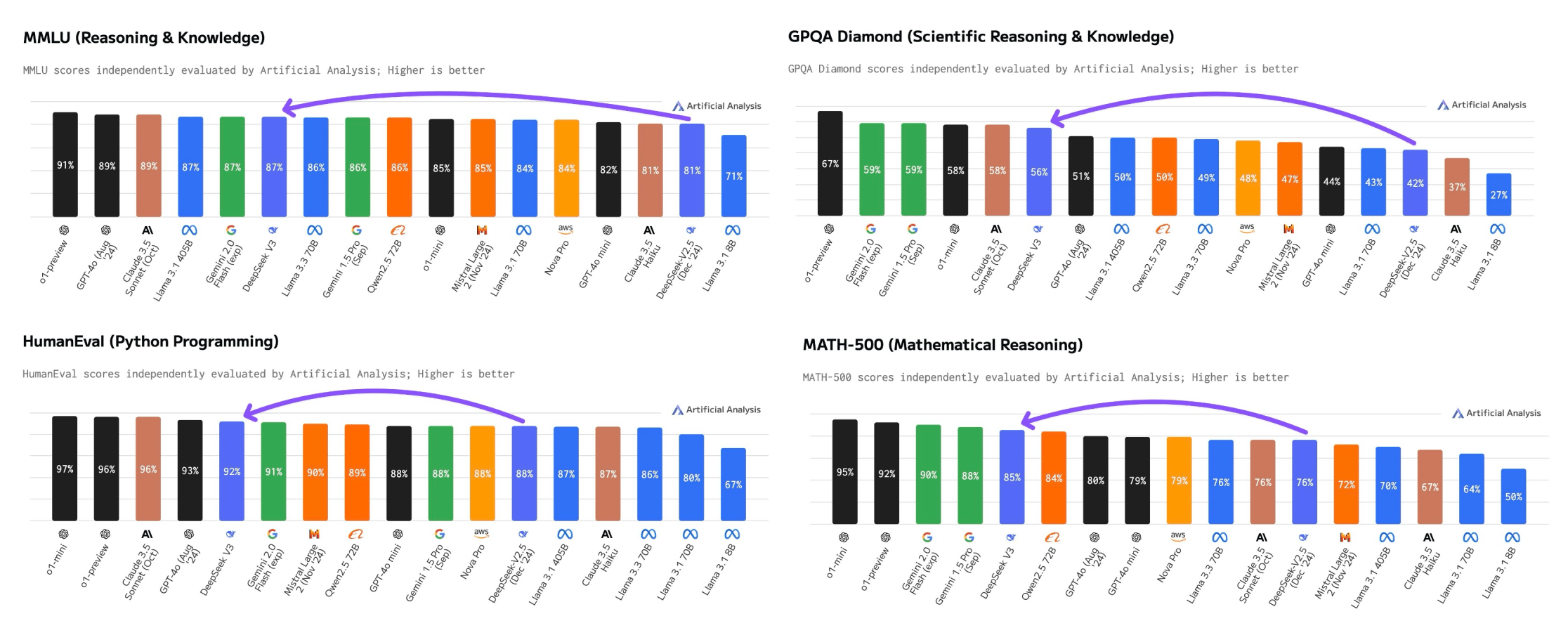
Of course, impressive benchmark scores don’t always mean a model will perform well in real-world situations. But the AI community is taking notice, particularly because Deepseek combines strong test results with unusually low training costs and has been completely transparent about their technical approach.
Doing more with less
The numbers tell a remarkable story about Deepseek’s efficiency. According to AI expert Andrej Karpathy, training a model this sophisticated typically requires massive computing power – somewhere between 16,000 and 100,000 GPUs.
Deepseek managed it with just 2,048 GPUs running for 57 days, using 2.78 million GPU hours on Nvidia H800 chips to train their 671-billion-parameter model. To put that in perspective, Meta needed 11 times as much computing power – about 30.8 million GPU hours – to train its Llama 3 model, which has fewer parameters at 405 billion.
Karpathy calls Deepseek’s budget “a joke” for a model of this caliber, highlighting how important resource efficiency has become. “You have to ensure that you’re not wasteful with what you have, and this looks like a nice demonstration that there’s still a lot to get through with both data and algorithms,” Karpathy writes. Despite these efficiency gains, he says, large GPU clusters will still be necessary for developing frontier language models
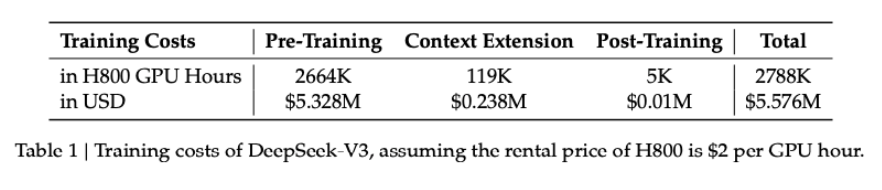
Part of Deepseek’s success comes from necessity. As a Chinese company facing U.S. export restrictions, they have limited access to the latest Nvidia chips.
Recommendation
The company had to work with H800 GPUs – AI chips designed by Nvidia with reduced capabilities specifically for the Chinese market. These chips have much slower connection speeds between GPUs compared to the H100s used in Western labs.
Deepseek turned this limitation into an opportunity by developing its own custom solutions for processor communication rather than using off-the-shelf options. It seems like a classic case of constraints driving creative problem-solving.
Price pressure
Deepseek’s lean operations and aggressive pricing strategy are forcing established players to take notice. While OpenAI continues to lose billions of dollars, Deepseek is taking a radically different approach – not only are they offering their best model at budget-friendly prices, they’re making it completely open source, even sharing model weights.
According to Artificial Analysis, while Deepseek V3 costs a bit more than OpenAI’s GPT-4o-mini or Google’s Gemini 1.5 Flash, it’s still cheaper than other models with similar capabilities. They offer a 90% discount for cached requests, making it the most cost-effective option in its class.
The company has raised prices from their previous version – doubling input costs to $0.27 per million tokens and increasing output costs fourfold to $1.10. But they’re softening the blow by keeping V3 at the old pricing until early February, and anyone can try it out for free on Deepseek’s chat platform.
Restrictions drive progress
Deepseek’s V3 shows an interesting consequence of US export restrictions: limited access to hardware forced them to innovate on the software side.
This could be particularly relevant for European AI development. Deepseek shows that building cutting-edge AI doesn’t always require massive GPU clusters – it’s more about using available resources efficiently. This matters because many advanced models don’t make it to the EU, as companies like Meta and OpenAI either can’t or won’t adapt to the EU AI Act.
But don’t expect data centers to go away anytime soon. The industry is shifting its focus to scaling inference time – the amount of time a model is given to generate answers. If this approach takes off, the industry will still need significant compute, and probably more of it over time.