Deepmind proves robust AI adaptation requires learning causal models under the hood
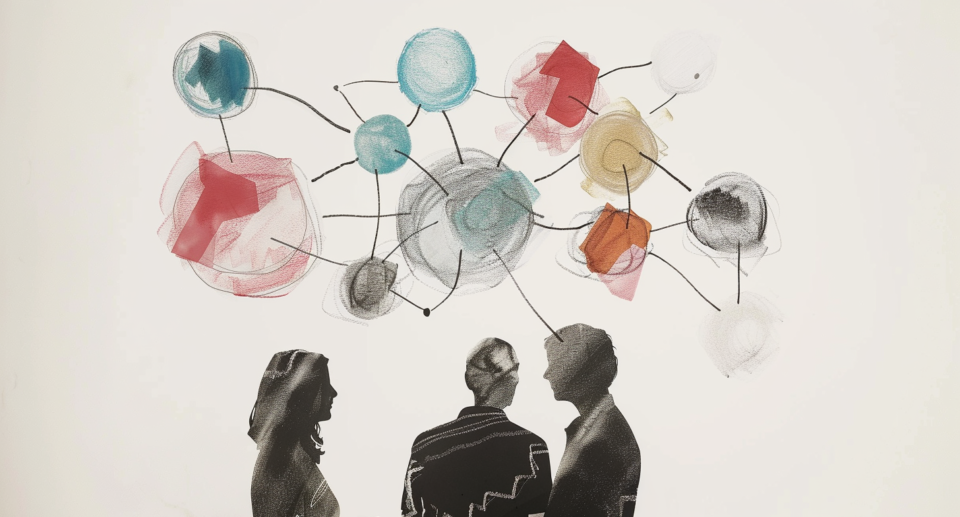
Researchers show that artificial intelligence must learn causal models to adapt robustly to new environments.
Learning causal relationships plays a fundamental role in human cognition. So is human-level AI impossible without causal reasoning? Recent advances in AI agents and models that can adapt to many environments and tasks without explicitly learning causal models challenge this view.
However, researchers at Google DeepMind have now mathematically proven that any AI system that can robustly adapt to changing conditions must necessarily have learned a causal model of the data and its relationships – just not explicitly.
In their study, they looked at decision tasks in which an AI agent must choose a strategy (policy) to achieve a goal. An example would be a classification system that needs to make a diagnosis based on patient data.
Ad
Ad
The researchers showed that if the distribution of the data changes (distributional shift), for example, because the agent is moved to a new clinic with different patient groups, the agent must adapt its strategy.
If the agent can do this with minimal loss, regardless of what changes occur in the data, then it must have learned a causal model of the relationships between the relevant variables, according to the mathematical derivation. The better the adaptivity, the more accurate the causal model.
The difference to explicit learning of causal models is that the AI learns these models, regardless of the method used to train it. Thus, the trained system contains knowledge about causal relations.
The researchers draw two conclusions from the theorems presented in the paper:
- Optimal policies encode all causal and associative relations.
- Learning to generalize under domain shifts is equivalent to learning a causal model of the data–generating process—problems that on the surface are conceptually distinct.
Causal models could explain emergent abilities
The results could, for example, explain how AIs develop so-called emergent capabilities: By training on many tasks, they learn a causal model of the world that they can then apply flexibly. However, according to the team, this requires that the causal model is recognizable from the training data.
Recommendation
“The question is then if current methods and training schemes are sufficient for learning causal world models. Early results suggest that transformer models can learn world models capable of out-of-distribution prediction. While foundation models are capable of achieving state of the art accuracy on causal reasoning benchmarks, how they achieve this (and if it constitutes bona fide causal reasoning) is debated.”
From the paper
The authors see their work as a step toward understanding the role of causality in general intelligence. Causal reasoning is the basis of human intelligence and may be necessary for human-like AI. Although some AI systems perform well without explicit causal modeling, the work shows that for robust decision-making, any system must learn a causal model of the data, regardless of training method or architecture. This model makes it possible to find the optimal strategy for each goal. The results point to a deep connection between causality and general intelligence and show that causal world models are a necessary component of robust and versatile AI.